My teammate and I lately did an interesting prediction project for one of our Business Analytics courses. This is an insurance firm that primarily provides health insurance to the public. Now they want to explore into the auto insurance segment. They have data on their existing customers which includes their age, area code, if they were insured previously, gender, vehicle age, premium cost, purchase channel, years stayed with company, and their willingness to purchase the car insurance, etc. This is a real dataset that contains 381,109 observations and 12 features, and it can be found from Kaggle. We use R as the programming language for this project.
Let's glimpse the dataset.
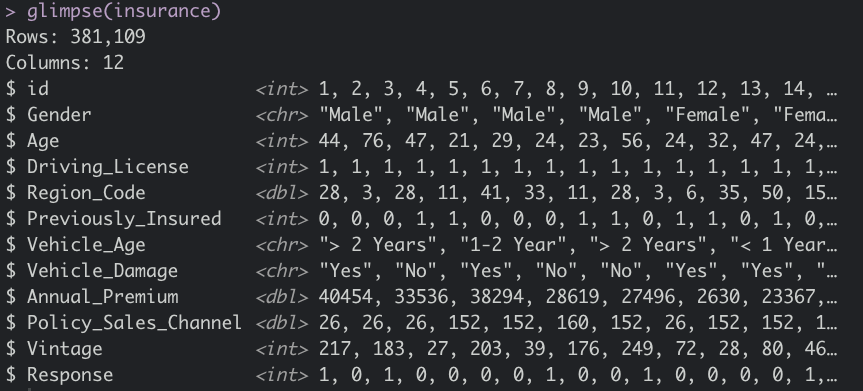
Business Problem
There is limited budget for the marketing operations. As a result, the management team wants to maximize return on capital. One major business problem is the need to understand which customer demographics are more willing to accept auto insurance offers if being targeted by a marketing campaign. This will ensure that marketing capital is not wasted on the customers that are not likely to respond to a marketing campaign. Another problem that the management would like to solve is the viability of cross-selling. They want to know if it is feasible to sell auto insurance to their existing customers.
Our Solution We implemented descriptive analytics methods to conduct a series of Exploratory Data Analysis (EDA) to extract useful information from the dataset. Then we did predictive analytics methods to identify the features that highly affect customers’ response rate to the new auto insurance offers. Also, we built prediction models for the response rate towards auto insurance offers based on the existing customers.
Findings
1.On average, only 12% of customers are willing to purchase the auto insurance

2. We can see that the majority customers are under 40 years old. But customers who are from 30 to 50 years old are more likely to purchase the insurance .

3. Customers who had damaged car records are more likely to purchase the insurance

4. Customers that previously were not insured are more likely to purchase

5. Customers whose car age is between one to two years are more likely to purchase
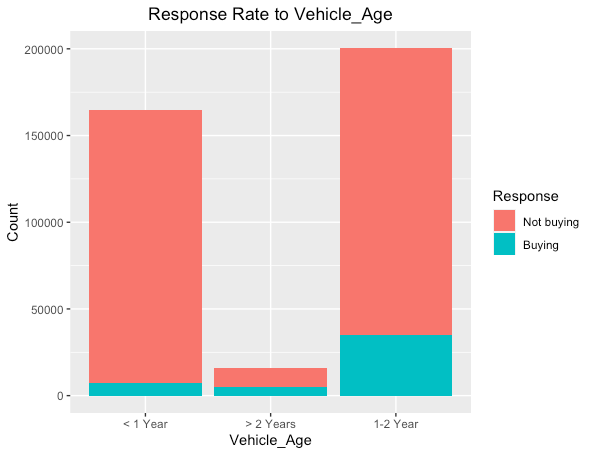
6. Customers who had premium plans between $30,000 and $40,000 are more likely to purchase
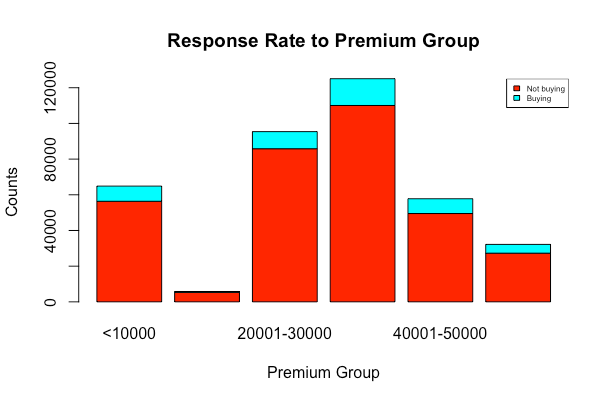
7. Sales channels in 3,4,26,31,124,154,155,156,157,163 had a higher proportion of customers who showed interest in purchasing auto insurance compared to other channels.
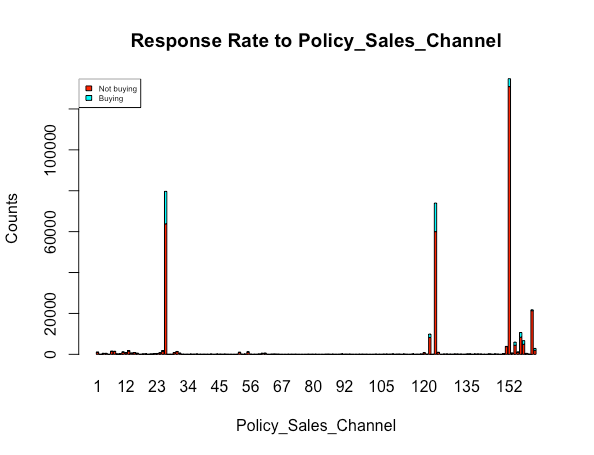
8. Male customers showed more interest in buying the insurance.
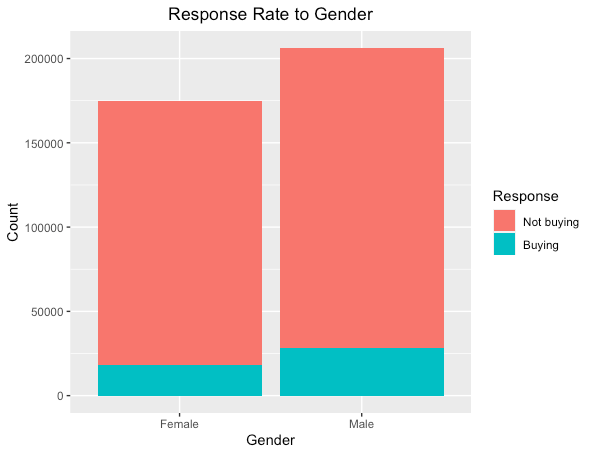
8. The correlation heatmap
From this heatmap, we can see that there is no very strong correlation between features. Most of them are under 0.5 score, suggesting they do have correlation, but not very strong.
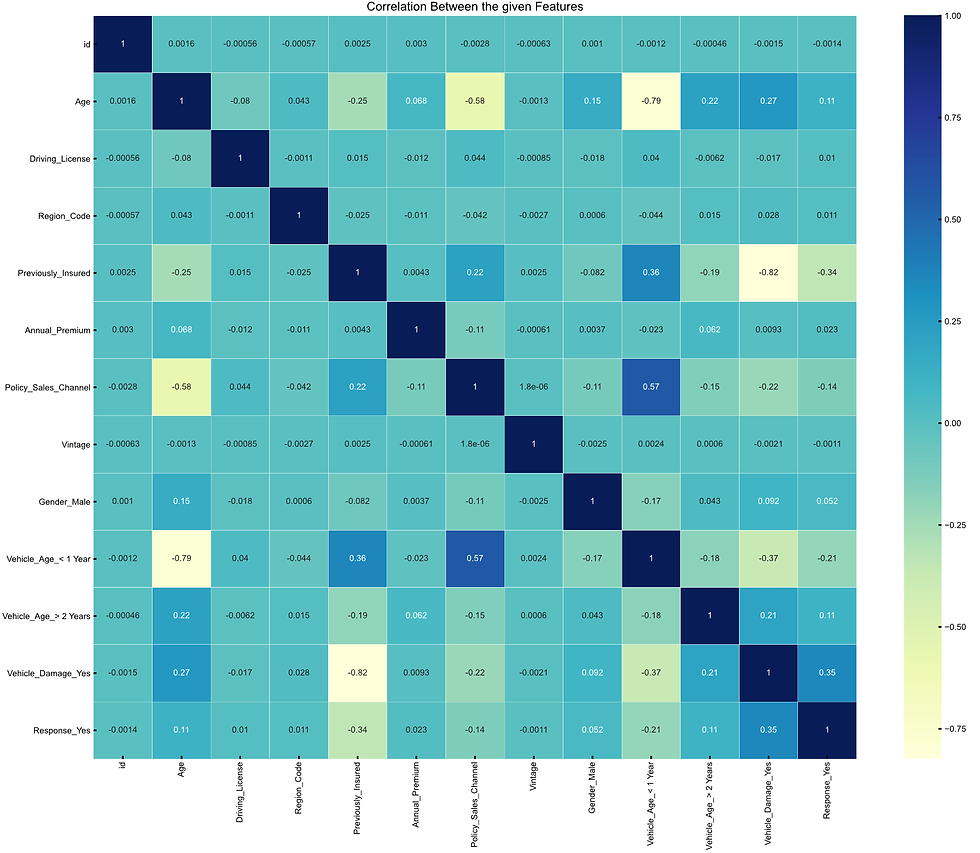
From the findings, should we think one of the possible target group of customers is "Older customers who had damaged car records and their car age was more than two years"? Or a male customer who had car damaged car record?
Methodology for Prediction
1.Logistic Regression In machine learning, this is a binary problem (Purchase or Not Purchase). Initially, we used normal Logistic Regression with all selected significant features, along with cross-validation for our prediction. However, this model performed poorly, though we received 87% accuracy. The recall rate was nearly 0 and precision rate was 1. We had extremely low true prediction for the Purchase class, extremely high false negative and zero false positive. The reason for this result was because we have imbalanced classes issue in this dataset. The ratio of Purchase and Not Purchase was 12/88. Hence, we next used L1 regularization with more advanced methods, such as resample and boosting to improve the classification accuracy. However, the Logistic Regression output did show us which variables are significant or not. Plus, by doing the interaction method, we can see that some variables together created interaction effect. This finding matches what we found from our EDA above.
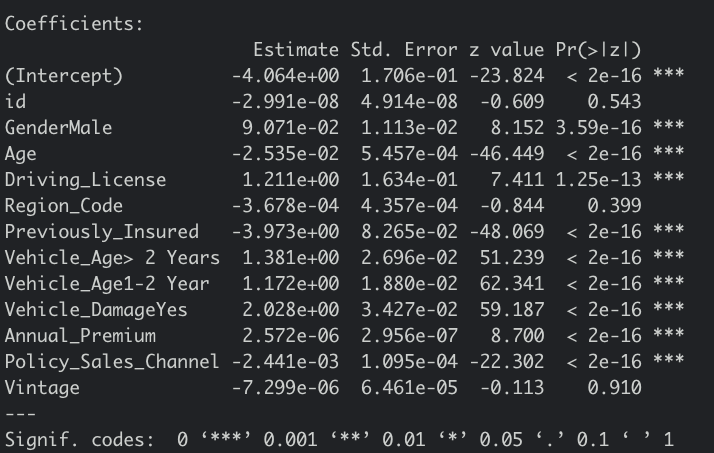

2. Lasso Regression L1 regularization is also called Lasso Regression, imposing a tune-penalty (0.0000000001) to regularize the coefficient estimates towards zero for the less contributive variables, thereby, keeping the most significant predictors in the model (kassambara,2018). We tuned the best lambda, used smote function for resampling. We received a lower accuracy rate, which was 64%, and high false positive rate, but a much higher prediction for the Purchase class. The recall rate was 97%, and the precision rate was 25%. However, this is still not our expected.
3. XGboost with GLM We used XGBoost with GLM model to improve our concerned problems---high false positive and low accuracy rate. XGBoost is a decision-tree-based ensemble Machine Learning algorithm that uses a gradient boosting framework (Morde,2019). Two benefits of using this model are time saving and performance improvement. We used different selected parameters and fit them to the model. This model gave us 87.48% accuracy, 8.6% recall rate, 46% precision rate and 98% specificity rate.
Business Recommendations
Cross-Selling Viability According to our models, 12% of the existing customers will consider purchasing the auto insurance. Marketing cost to acquire these customers are fairly low due to their existing brand loyalty. Afterall, the cost to acquire completely new customers is much higher than to acquire current customers. The firm could target them on the firm app, website, or by sending emails. We recommend the firm to pursue the current cross-selling strategy.
Response Prediction The XGBoost model will gave us 87.48% accuracy, 8.6 recall rate ,46% precision rate and 98% specificity rate. The AUC is 0.86, which is fairly good. It is not easy to have a high recall and precision rate with this dataset due to imbalanced class issue. However, we can predict 98% customers will not purchase the insurance, indicating the false positive rate is low. But we could actually adjust the cutoff value to receive either higher or lower specificity rate. This model at least will not waste marketing budget. Compared to missing profit, not wasting marketing budget is more important in this cross-selling opportunity. Thus, our model would aid the management in deciding marketing and communication strategies at a certain level.
Important Customer Features Age According to our analysis, people from 31 to 40 and 40 to 50 years old are more likely to respond to the auto insurance offers. We can target these two groups of people through the appropriate marketing channels. Make sure to implement services and use languages that are appropriate for these demographics.
Car Damaged Record Customers that had damaged car records are more willing to accept the auto insurance offers. Intuitively speaking, people who have suffered from an accident know the negative impacts of it on their life. Thus, educating customers of the danger of car accidents might improve response rate. 1 in 4 Americans say COVID-19 has increased their interest in life insurance (PR Newswire, 2020). The same logic could be applied to auto insurance.
Premium plan Customers who had premium plans between $30,000 and $40,000 are more likely to accept the auto insurance offers. Both auto insurance and health insurance might be needed in a car accident (Lynch, n.d.). Customers might find these plans don’t offer enough coverage, so they are more inclined to pursue additional coverage through auto insurance.
Previous insurance Customers who were not previously insured are more likely to accept the offers. It is possibly because their current plans can’t satisfy their needs, so extra coverage is required. While customers who were previously insured showed extremely low interest in the offer. This is possibly because they either already have an auto insurance or don’t need it at all. Company can keep this group of people on a waiting list, don’t put them as the first target customers. Otherwise, they could be the sleeping dogs, indicating that they could just leave the company entirely if the company pushes them too much.
Age of Vehicle Customers that have vehicle age between one and two are of our interest. However, this category is quite vague since the average vehicle age is 11.9 years (Beresford, 2020). We recommend the firm to gather more information for this category.
Sales Channels From the above section, we found 10 channels that were highly correlated with high customer response rates compared to other channels. We can have the marketing campaign specifically target these channels. Moreover, meetings with the appropriate personnel in those channels will be necessary in order to understand the reason for the higher willingness to purchase. We will be able to gain more insightful information from these meetings that will eventually aid our marketing campaign.
We recommend contacting customers during the health insurance enrollment period to check their satisfaction of the current plan. During the conversation, we can ask whether the cost is manageable, how the coverage is working, and how the current plan can be improved. From the answers to these questions, we can know that if the customers can afford a supplemental coverage, or if the auto insurance is right for them.
Building Strong Customer Relationship We recommend targeting those who have stayed with the firm for an extended period of time. Targeting the just-in new customers for cross-selling might cause them to lose interest. Intuitively speaking, if I just joined the health insurance company for less than three months, and the company tries to sell me other products, I would think that they just want to make more money from me. It is vital to establish brand loyalty and trust with the customers first before introducing new products. Therefore, we recommend selling to the customers who have stayed in the company for at least three months. As senior consultants at Bain & Company point out, customer churn in the insurance industry drops sharply as an insurer sells customers another one or two products (Sherer, Springer & Senior, 2016). That’s why Customer Relationship Management (CRM) system is popular today. Having solid relationship with customers, the sales opportunities will naturally come.
Further Actions Priority life events that can trigger the purchase of a new vehicle are reliable predictors of insurance purchases. Customer behavior data is missing from the current dataset. Behavior data would greatly aid in the prediction capabilities of our models (Ethan Jacobs, 2020). We recommend the firm to gather more behavior data on their customers. Specifically, whether their customers compare prices, look for discounts, and how many times they’ve received offers from other insurance firms.
References: Beresford, C. B. (2020, November 10). Average Age of Vehicles on the Road is Approaching 12 Years. Car and Driver. https://www.caranddriver.com/news/a33457915/average-age-vehicles-on-road-12- years/#:%7E:text=A%20study%20from%20IHS%20Markit,month%20older%20than%20in%202019. Ethan Jacobs, E. J. (2020, November 18). Car Insurance Shopping Behavior Survey. Reviews.Com. https://www.reviews.com/insurance/car/shopping-behavior-survey/ Gyant, N. G. (2018, April 14). How to Market Effectively to an Older Demographic. ThriveHive. https://thrivehive.com/how-to-market-effectively-to-an-older-demographic/ Kumar, A.(n.d.). Health Insurance Cross Sell Prediction. Kaggle. Retrieved from https://www.kaggle.com/anmolkumar/health-insurance-cross-sell-prediction Morde, V.(2019, April 7). XGBoost Algorithm: long may she reign!. Towardsdatascience. Retrieved from https://towardsdatascience.com/https-medium-com-vishalmorde-xgboost-algorithm-long-she-may- rein-edd9f99be63d Quick-R: Tree-Based Models. (n.d.). Quick-R. Retrieved December 15, 2020, from https://www.statmethods.net/advstats/cart.html Senior, J., Springer,T. & Sherer, L. ( 2016, October 11). Reinvigorate cross-selling. Bain & Company. Retrieved from https://www.bain.com/insights/reinvigorate-cross-selling/ PR Newswire. (2020, May 28). 83 Million Americans Say COVID-19 Makes Them More Likely to Buy Life Insurance. PR Newswire. https://www.prnewswire.com/news-releases/83-million-americans-say-covid- 19-makes-them-more-likely-to-buy-life-insurance-301066917.html Lynch, A.L. (n.d.) Car Insurance vs. Health Insurance: A Guide, from https://www.thezebra.com/auto- insurance/insurance-guide/car-insurance-vs-health-insurance/
Comments